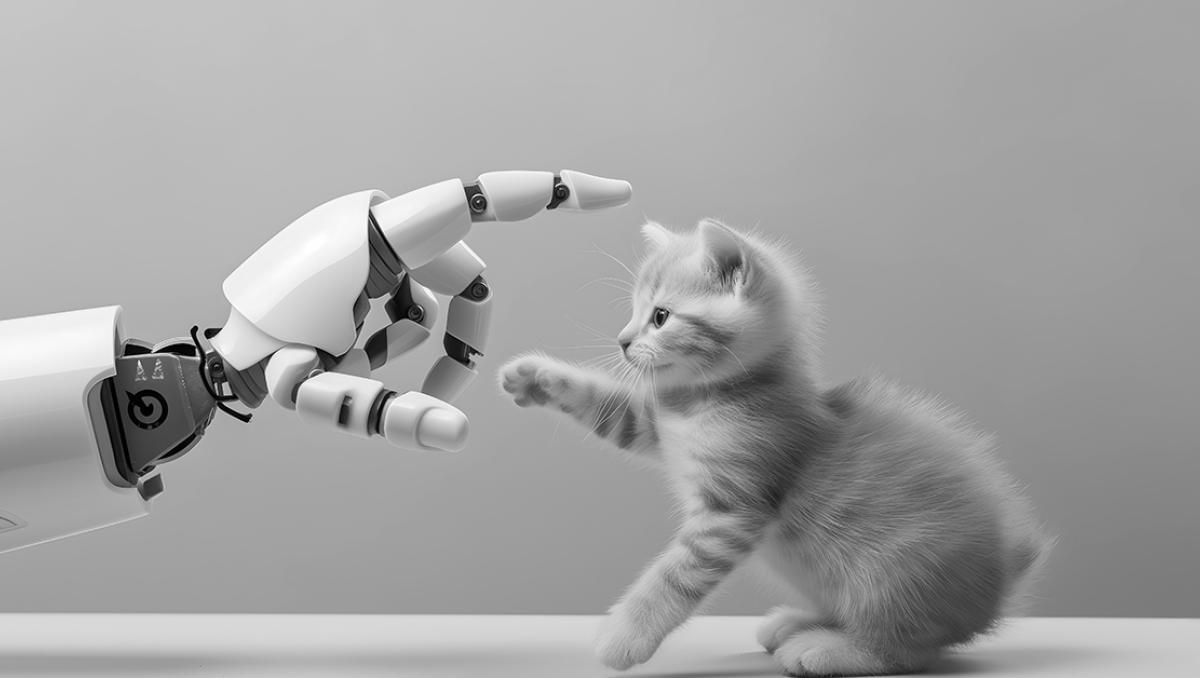
It has been only one year and nine months since OpenAI made ChatGPT available to the public, and it has already had a massive impact on our lives. While AI will undoubtedly reshape our world, the exact nature of this revolution is still unfolding. With little to no experience, security administrators can use ChatGPT to rapidly create Powershell scripts. Tools like Grammarly or Jarvis can turn average writers into confident editors. Some people have even begun using AI as an alternative to traditional search engines like Google and Bing. The applications of AI are endless!
Generative AI in Cybersecurity – The New Gold Rush?
Fueled by AI’s versatility and transformative potential, a new gold rush is gripping businesses across all industries. From healthcare and finance to manufacturing and retail, companies are scrambling to stake their claim in this technological frontier. The adoption of generative AI in cybersecurity is accelerating, with many companies actively adding or having already added these capabilities to their platforms. But it presents an important question – are we doing too much too soon?
I recently attended a think tank where the primary topic was Generative AI in Security. The event started with a vendor and their MSP partner showcasing how the vendor’s generative AI capabilities are helping the MSP streamline threat mitigation for their clients. They boasted significant time savings, resulting in the MSP optimizing their analyst team. Notably, they’ve adjusted their hiring strategy from recruiting seasoned professionals to extending opportunities to junior analysts while leveraging AI to assist with training and guiding the new analysts, potentially accelerating their path to proficiency in cybersecurity. They also boasted how they reduced their analyst staff from 11 to 4. The reduction of operational overhead led to reduced costs for both the MSP and their clients. There are many pros and cons to that statement. The impact of AI on existing jobs is a topic best left for another time, as the full extent of its positive job creation potential remains unknown.
To What Extent Can We Trust AI?
Discussions on trust and generative AI often focus on who owns the data users provide, how the data users provide helps train AI models, and AI’s ability to share and recommend proprietary data with other users. A critical aspect often neglected is the significant threat posed by inaccurate data.
I recently suggested my son use ChatGPT to break down the order of operations for his math homework. After a couple of hours, he said he still couldn’t solve the problem. I sat down with him to review the advice AI provided, and while the answer was well articulated and beautifully formulated, it was far from accurate. The poor kid was spinning himself in circles using a flawed method to solve a math problem. That situation immediately came to mind when the executive from the MSP explained their reliance on generative AI to guide junior security analysts.
Two critical questions emerge concerning Generative AI: who is responsible for ensuring the accuracy of its data, and who bears the liability for any consequences arising from inaccurate outputs?
According to Google’s Gemini, data accuracy in AI is a shared responsibility, with different players involved.
- Data Providers: These entities collect and supply the data used to train AI models. Their responsibility is to ensure the data they provide is accurate, complete, and unbiased.
- AI Developers: The developers who design and train the AI models have a role in assessing the quality of the data they use. They should clean and pre-process the data to minimize errors and identify potential biases.
- AI Users: Those who deploy and utilize the AI models also share some responsibility. Understanding the limitations of the model and the data it was trained on is crucial (we need transparency in this area).
The answer on liability was not as clear. There isn’t always a single party held responsible. Depending on the jurisdiction and the specific use case, there may be legal and regulatory requirements that dictate liability, but the legal landscape for AI liability is still developing and will likely evolve as more incidents and case law emerge.
Looking at the Past to See the Future:
Examining the past can often provide insights into the future. The potential of AI may draw some similarities to the history of search engines. Google’s page rank methodology is a great example. The algorithm significantly improved search result relevance. Personalization and location features furthered user benefits. However, the addition of personalization led to unintended consequences like the filter bubble, where users only encounter information that reinforces their beliefs. SEO manipulation and privacy concerns have also affected the benefits and relevancy of search engines.
Similar to how search engines can struggle with bias, generative AI models trained on massive datasets can also reflect these biases in their outputs. Both platforms will be a battleground for misinformation, making it difficult for users to discern truth from falsehood. In either case, users should always validate the accuracy of the results. From a personal and business standpoint, any person who is using generative AI should create a process to validate the information they receive. One thing I like to do is ask the AI to provide reference links to the sources where it pulled the information provided in its answer. Depending on the subject, I might even check other sources.
Another aspect that affected search relevance is advertisements. While I don’t think Generative AI tied into cybersecurity platforms will include advertisements, I can foresee a world where the Generative AI platform is upselling and cross-selling other products. Want to enhance visibility? Try out our or our partner’s new widget. Another factor to consider is whether AI will be able to identify their technology as the issue, and if so, will it tell you that?
Ending Note
If you’re using AI to build a macro-based diet plan or guide your cybersecurity posture, it’s vital to maintain awareness of its faults and limitations. Always use critical thinking when evaluating AI outputs, and never base your decisions or inputs solely on the information it provides.
Living in this age of AI feels like a thrilling rollercoaster ride – exhilarating, full of potential, but also a bit nerve-wracking. While the future holds immense promise, it’s crucial to ensure we’re strapped in securely. Transparency from providers and a robust regulatory framework from lawmakers are essential safeguards. These measures will help us navigate the twists and turns, minimizing risks and maximizing the benefits of AI. However, a lingering concern remains. Are we pushing the envelope too quickly? Open dialogue and collaboration between developers, users, and policymakers are vital. By working together, we can establish responsible practices and ensure AI becomes a force for positive change, not just a wild ride.
To learn more about the challenges and opportunities of generative AI with Fortra, you can read this blog by Antonio Sanchez.