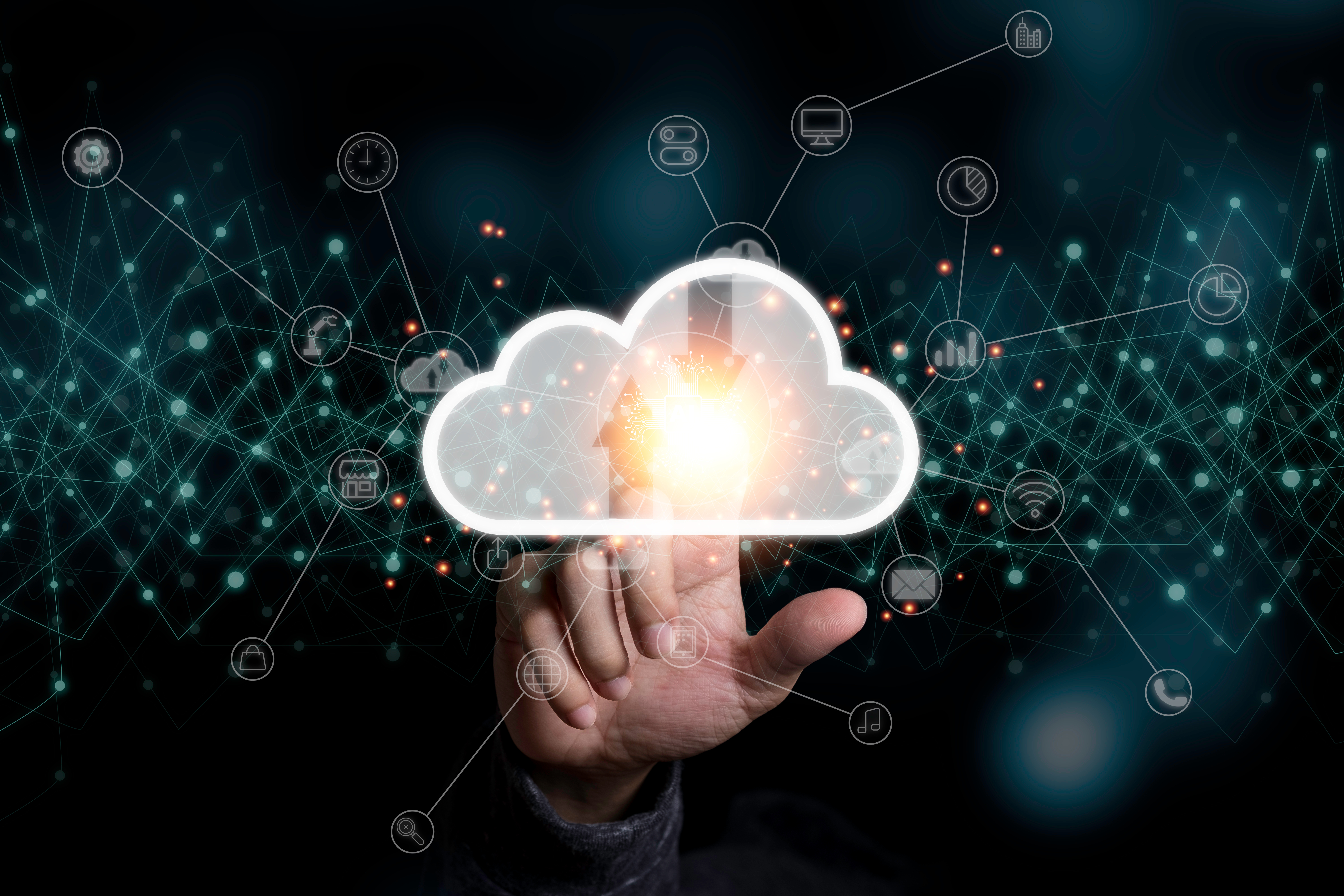
We’re on the cusp of an artificial intelligence revolution, and the generative AI trend doesn’t seem to be slowing down anytime soon. Research by McKinsey found that 72% of organizations used generative AI in one or more business functions in 2024—up from 56% in 2021.
As businesses explore how generative AI can streamline workflows and unlock new operational efficiencies, security teams are actively evaluating the best way to protect the technology. One major gap in many AI security strategies today? Generative AI workloads.
While many are familiar with the mechanisms used to secure AI models like OpenAI, ChatGPT, or Anthropic, AI workloads are a different beast altogether. Not only do security teams have to assess how the underlying model was developed and trained but they also have to consider the surrounding architecture and how users interact with the workload. In addition, AI security operates under a shared responsibility model that’s similar to the cloud. Workload responsibilities vary depending on whether the AI integration is based on Software as a Service (SaaS), Platform as a Service (PaaS), or Infrastructure as a Service (IaaS).
By only considering AI model-related risks, security teams miss the bigger picture and fail to holistically address all aspects of the workload. Instead, cyber defenders must take a multilayered approach by using cloud-native security solutions to securely configure and operate multicloud generative AI workloads.
How layered defense secures generative AI workloads
By leveraging multiple security strategies across all stages of the AI lifecycle, security teams can add multiple redundancies to better protect AI workloads—plus the data and systems they touch. It starts by evaluating how your chosen model was developed and trained. Because of generative AI’s potential to create harmful or damaging outputs, it must be responsibly and ethically developed to guard against bias, operate transparently, and protect privacy. In the case of companies that ground commercial AI workloads in proprietary data, you must also ensure the data is of a high enough quality and sufficient quantity to produce strong outputs.
Next, defenders must understand their workload responsibilities under the AI shared responsibility model. Is it a SaaS-style model where the provider secures everything from the AI infrastructure and plugins to protecting data from access outside of the end customer’s identity? Or (more likely) is it a PaaS-style arrangement where the internal security team controls everything from building a secure data infrastructure and mapping identity and access controls to the workload configuration, deployment, and AI output controls?
If these generative AI workloads operate in highly connected, highly dynamic multicloud environments, security teams must also monitor and defend every other component the workload touches in runtime. This includes the pipeline used to deploy AI workloads, the access controls that protect storage accounts where sensitive data lives, the APIs that call on the AI, and more.
Cloud-native security tools like cloud security posture management (CSPM) and extended detection and response (XDR) are especially useful here because they can scan the underlying code and broader multicloud infrastructure for misconfigurations and other posture vulnerabilities while also monitoring and responding to threats in runtime. Because multicloud environments are so dynamic and interconnected, security teams should also integrate their cloud security suite under a cloud-native application protection platform (CNAPP) to better correlate and contextualize alerts.
Holistically securing generative AI for multicloud deployments
Ultimately, the exact components of your layered defense strategy are heavily influenced by the environment itself. After all, protecting generative AI workloads in a traditional on-premises environment is vastly different than protecting those same workloads in a hybrid or multicloud space. But by examining all layers that the AI workload touches, security teams can more holistically defend their multicloud estate while still maximizing generative AI’s transformative potential.
For more insight into securing generative AI workloads, check out our series, “Security using Azure Native services.”